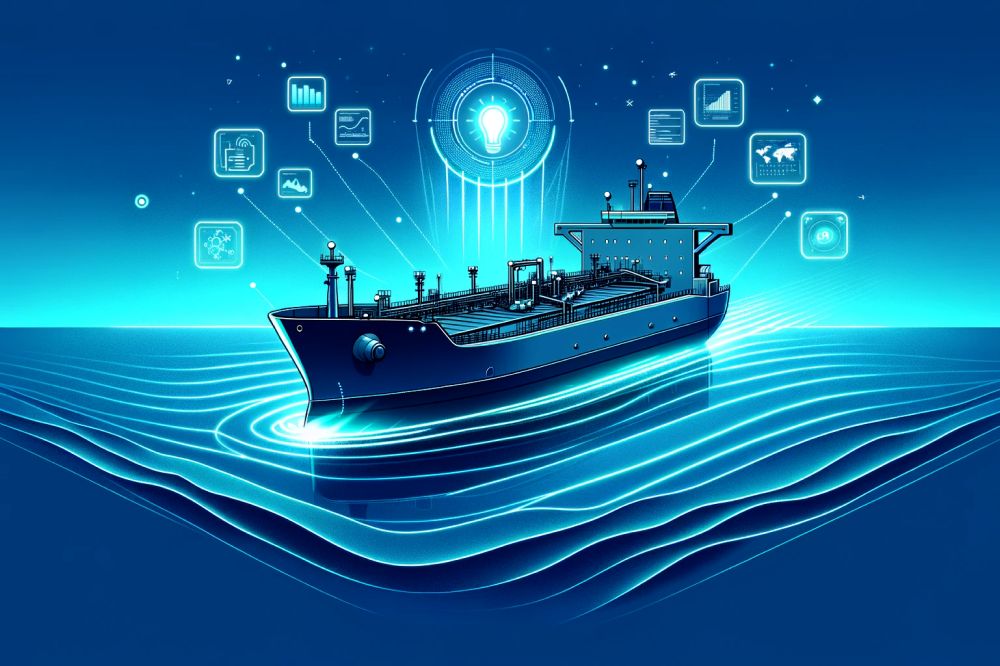
Generative AI will unlock a wide range of new capabilities for companies involved in maritime business
Generative AI technology is advancing rapidly and will unlock a wide range of new capabilities. However…
Generative AI will not generate magic unprompted, and it will only create value for those who possess the knowledge to effectively leverage these AI agents because they possess an understanding of the context these AI agents operate under, have solid industry knowledge and understanding of its company’s business models.
McKinsey’s interviewed their senior partner Jorge Amar about the AI potentials and hurdles. He says that generative AI agents can and will transform various corporate services and workflows. It will help automate a lot of tasks that are not adding value while creating a better experience for both employees and customers. To carve out these potentials, change management will be critical. That’s a big hurdle for organizations. It will be much broader than simply “rolling out a new set of tools”. Companies are going to need to rewire how functions work so they can get the full value from gen AI agents.
Mr. Amar is also worried about the quality of the data, which needs to be of high quality and in the right format for gen AI technologies to use them effectively. Almost 20% of most organizations see data as the biggest challenge to capturing value with gen AI.
AI agents can and will transform various services and workflows offered by companies and the changes will happen over time, but you should get onboard today to prepare and learn.
But how will these new technologies influence the maritime industry?
I had a conversation with my new CoPilot friend and asked it some questions about maritime digitalization and AI, which I want to share with you;
Me: What are the challenges of implementing AI in the maritime industry
We have heard it before, lack of standards and definitions and machine-readable formats. In Maritime Optima we encountered these problems from the get-go, when starting the company in 2019. Whereas developers require definitive answers to logical questions, the maritime industry often provides the opposite.
“What do you mean when you say Flag of Convenience, isn’t a flag a flag and belong to a nation?”, "Why is it so many abbreviations for Rotterdam?","Why haven’t this industry spent time on defining data formats when there are so many softwares available?"
So, it is actually back to basic… machines prefer to work with structured data that are easy for machines to read and understand and interpret.
We've heard this one before as well. New technology, and AI in particular, require very costly investments. Furthermore, they need to be taken up front because building a digital data infrastructure is needed before gaining any digital value.
If you are lacking the competence and see a huge investment coming your way, in something you don’t understand, are you willing to invest?
Yeah, cyber security can be used as an excuse for not doing anything (continue as before and close the bars) and for not sharing data. Several software companies will tell you about all the pitfalls you can enter and must avoid them, to sell their advises and consultancy services.
Nothing new once again. The right competence and talents have always been important. In my previous career I did a report on “How to attract talents to Norway”, and the answer is not always a quick one. All universities will tell you, and all management programs will reconfirm, that the need for the right talents, the right skills and the right knowledge, is of utmost importance for any type of company. The automation potential of AI raises concerns about job displacement and make experienced employees’ resistant to AI adoption due to fear of unemployment. It is of utmost importance to emphasize that AI can augment human capabilities and create new roles rather than replace jobs. It can help with automizing nonproductive and boring workflows.
So, addressing these challenges and making the maritime industry fitted for AI requires a global collaborative effort from the industry stakeholders, policymakers, and technology providers. Implementing AI solutions requires significant upfront investment in technology, infrastructure, and skilled personnel. Technology seems to have an unhidden muscle and become better and stronger every day. However, this will take years, but if we can overcome these obstacles, it will pave the way for AI-driven advancements in the maritime sector.
How can SMBs participate in the AI race?
Is there no other way to get started with digitalization than to wait for years until global policy makers and IMO agree? Are there any other alternatives?
How can SMBs prepare to participate in this AI race if the upfront costs are so high, skilled people are hard to get, the data is unstructured, and there is a lack of willingness to share data between vendors and software providers? There are thousands of SMB companies involved in maritime business around the globe. Will they be able to take place in this AI race? Or will all the benefits only be available for the large enterprises?
First remember; after all, digital transformation isn’t about adopting every technology available. It’s about strategically using technology to improve processes, enhance customer experiences, and stay competitive in a rapidly evolving landscape.
We believe that investing in big data technologies capable of storing and processing the volumes of data required by AI technologies can serve as a catalyst for unlocked its true potential. Let’s start by defining a couple of different types of storage options such as Data Warehouses, Data Lakes and Data Lakehouses.
Below is a table from striim.com and it quickly summarizes the differences between the data warehouse vs. data lake and the data lake house:
Source: striim.com
Data warehouses
A data warehouse is a unified data repository for storing large amounts of information from multiple sources within an organization. The disadvantages of data warehouses are that they prefer structured data, and struggle with semi-structured and unstructured data formats which can be used for machine learning and AI use cases. Also, the costs for implementation and maintenance are very high.
Data lakes
Data lakes are particularly useful for managing high-volume, varied, and rapidly arriving data. They can include structured data (such as rows and columns from relational databases), semi-structured data (like CSV, logs, XML, and JSON), unstructured data (such as emails, documents, and PDFs), and even binary data (like images and audio files). These repositories empower data scientists, developers, and analysts to extract valuable insights from vast amounts of information.
Data lakes’ lack of data consistency makes it difficult to enforce data reliability and security. Because data lakes can accommodate all data formats, it might be challenging to implement proper data security and governance policies to cater to sensitive data types. Data lakes offer flexibility and affordability; however, it is essential to implement proper data governance practices to avoid turning them into "data swamps".
A data lakehouse
The data lakehouse is the newest data storage architecture that combines the cost-efficiency and flexibility of data lakes with data warehouses’ reliability and consistency.
According to Striim.com the main disadvantage is that it is a new and immature technology, so it might not live up to its promises.
It may be years before data lakehouses can compete with mature big-data storage solutions. But with the current speed of modern innovation, it’s difficult to predict whether a new data storage solution could eventually usurp it.
- Scalability: Data lakehouses can handle large volumes of data, making them suitable for the maritime industry, which generates vast amounts of information. Scalability ensures that as more data becomes available, the system can accommodate it seamlessly.
- Generative AI Benefits: Once data is stored in a data lakehouses, generative AI models can leverage it for various tasks. These models can learn patterns, generate synthetic data, improve predictions, and enhance decision-making. For example, generative AI can create realistic ship designs, optimize routes, or predict maintenance needs.
- Data Governance: While data lakes and data lakehouses offer flexibility, proper governance is crucial. Organizations must define policies for data quality, security, and access control. Implementing metadata management ensures that data remains discoverable and understandable.
Data lakes and data lakehouses provide a must-have foundation for leveraging generative AI in the maritime industry. By centralizing data, accommodating diverse sources, and maintaining flexibility, organizations can unlock the benefits of AI-driven insights and innovation with programming skills, domain expertise and willingness to invest. It can work with unstructured data, but the more structured the data is, the higher the volume of data, the more value you get out of it and the more affordable it will be to maintain the data structure, the data quality and to run inquiries.
Are data lakes and data lakehouses worth the investment for SMBs?
Is vast data storage technologies such as data lakes and data lakehouses the catalysts that will sort the lack of structured data, costs and lack of knowledge?
Both yes and no, they can help with the challenges posed by the lack of standardized data across the various maritime systems and platforms, but they come at a cost and skilled personnel is needed.
Should you dare to invest in such digital infrastructure in-house?
As a SMB company, probably not since it might become very costly setting up and using a data lake and data lakehouses. Here are some considerations:
- Infrastructure Costs: Data lakes require storage resources. Big data storage might not be so costly, but the cost depends on the amount of data stored and the chosen storage provider.
- Data Ingestion and Processing: Ingesting data into the data lake involves costs related to data transfer and processing can be costly. For example, if you’re ingesting large volumes with high velocity, it may be very costly.
- Data Governance and Security: Implementing proper data governance (metadata management, access controls, compliance) adds costs. Security measures to protect data also contribute to implementation costs.
- Data Lake and Data Lakehouse tools and Services: Costs vary based on the tools and services you use.
Regular maintenance, monitoring, and optimization are needed, and efforts contribute to ongoing costs. This includes managing schema evolution, performance tuning, and ensuring data quality. As this infrastructure grows, scaling resources (storage, compute) may increase costs. However, the flexibility to scale as needed is a must and will benefit your organization.
Summing up data lakes and data lakehouses:
The new data technologies offer flexibility and scalability; however, organizations should carefully assess their specific requirements and budget constraints.
Hiring experts and training staff to manage and utilize the data lake and data lakehouses effectively might be a struggle, in addition to having the tech competence and skills they should also have domain knowledge, so the question will be; how many persons are there in the world with solid maritime domain knowledge, backend and data science skills?
Proper planning, cost estimation, and optimization strategies are essential for successful data lake and data lake house implementations. So basically… costs estimates are difficult to make but if you want to build this in-house, you should expect it to be very costly.
So how can SMBs prepare to participate in this AI race?
Small and medium-sized businesses (SMBs) can strategically take part in digitalization without overspending on in-house technologies.
- Embrace Cloud Solutions: Leverage cloud computing to reduce infrastructure costs and capital expenditures. Cloud services offer scalability, flexibility, and pay-as-you-go models, allowing SMBs to access advanced technologies without heavy upfront investments.
- Prioritize Digital Transformation: Develop a clear digital transformation strategy. Identify areas where technology can enhance efficiency, productivity, and customer experience. Focus on personalized solutions that align with your business goals.
- Automate Routine Tasks: Use automation to streamline repetitive processes. By reducing manual effort, you can save time, minimize errors, and allocate resources more effectively. Automation also enhances consistency across touchpoints.
- Data-Driven Decision Making: Invest in data analytics tools. Analyzing data helps SMBs understand customer behavior, optimize operations, and identify growth opportunities. Data-driven insights drive informed decisions.
- Collaborate and Share Information within your team: Make everyone contribute to collection data. Break down silos within your organization. Implement digital tools that centralize information, ensuring everyone has access to a single source of truth. Collaboration tools enhance communication and efficiency.
- Start Small and Scale: Begin with manageable digital initiatives. Pilot projects allow you to test solutions, learn from feedback, and iterate.
How do you get started?
Let’s ask ChatGPT:
Me: Do you think ShipIntel by Maritime Optima can help maritime SMB companies with participating in the digitalization in the maritime industry without overspending on in-house technologies?
In summary, ShipIntel offers an alternative to costly in-house architecture and technologies, empowering SMBs to participate in maritime industry digitalization.
Instead of building all the infrastructure you need yourselves, you can start using the infrastructure Maritime Optima has built, accessing a digital infrastructure at almost no cost.
If you want to get access to a low cost – high secured maritime AI solution, you should integrate your ShipIntel subscription with your Microsoft 365 subscription, giving you the best of the best; public data (e.g. AIS), your private data (emails and documents etc) and maritime trained AI bots.
ShipIntel is made by maritime professionals for maritime professionals, to help any type and size of company take part in the maritime digitalization.
Let us show you what ShipIntel can do for you, book a demo today.